Optimized DL Ensemble Boosts Early Breast Cancer Detection
Enhancing Early Breast Cancer Diagnosis Through Automated Microcalcification Detection Using an Optimized Ensemble Deep Learning Framework
Tags: Universiti Malaya, Malaysia, Computing Technology, Healthcare & Lifesciences
This study introduces an optimized ensemble deep learning framework for detecting microcalcifications in mammograms, significantly enhancing early breast cancer diagnosis. By combining models like AlexNet, ResNet-50, and GoogLeNet, the framework improves accuracy, precision, and reliability. The ensemble model's ability to distinguish microcalcifications outperforms individual models, achieving high predictive confidence. This technology has applications in clinical settings, improving early breast cancer detection and patient outcomes. The ensemble approach demonstrates superior classification performance across key metrics such as F1-score and AUC.
IP Type or Form Factor: Discovery & Research; Software & Algorithm
TRL: 4 - minimum viable product built in lab
Industry or Tech Area: Big Data Analytics & Simulations; Diagnostics & Screening
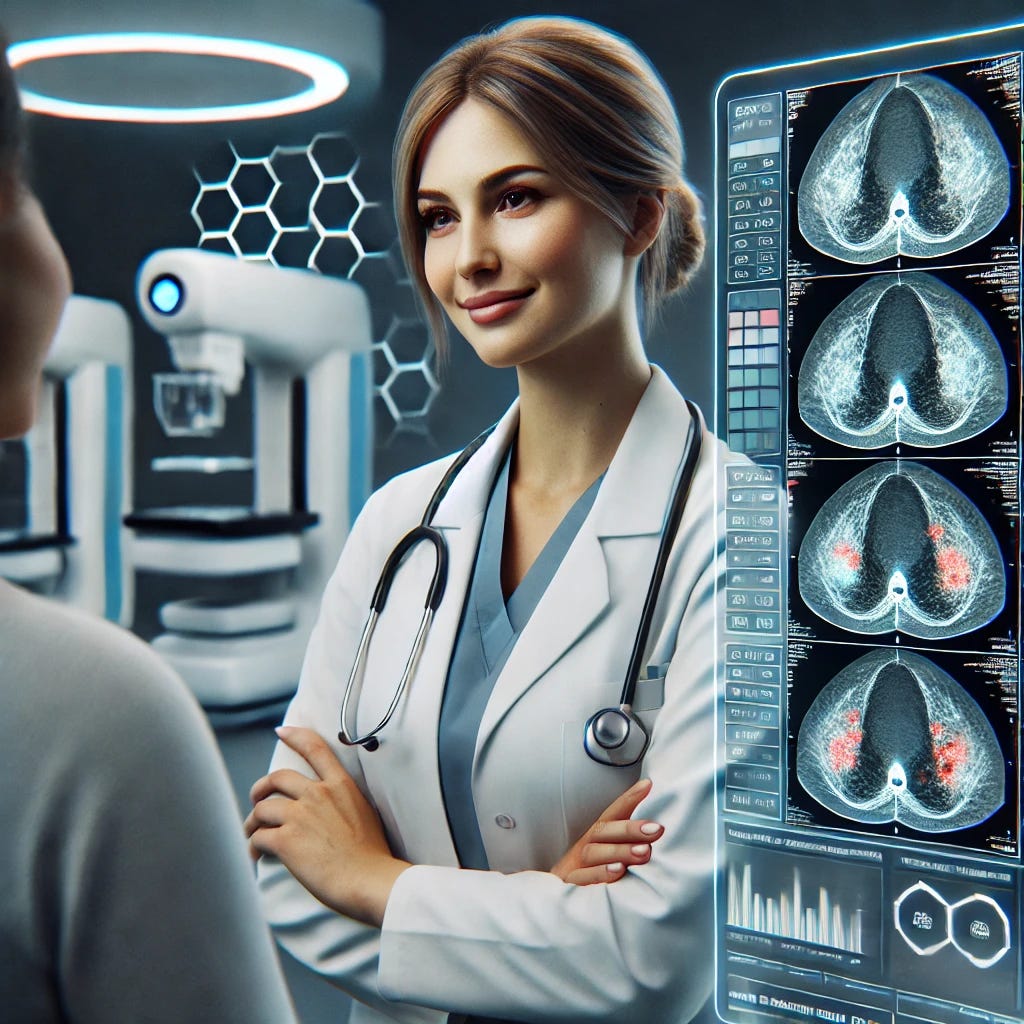